Category: News
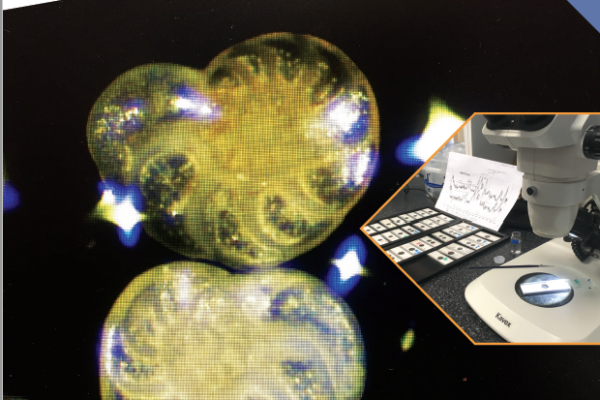
Annual Review 2023
Read More
Project Title: Novel statistical models and efficient inference methods for complicated ecological data.
The loss of biodiversity has been growing rapidly over the past few decades, with thousands of animal species now being threatened with extinction. Assessing the extent of ecological risks imposed on animal populations and developing appropriate interventions to support the species conservation require an adequate understanding of the population characteristics, such as population size or survival. Capture-recapture (CR) methods have been used extensively to estimate these characteristics. CR data are collected by sampling animals in the population over time, for example via DNA samples from animal hairs or feathers, photos of animals, or their natural marks. Most statistical models for CR data in the literature rely on the assumption that all captured individuals are correctly and uniquely identified, however assuming this is not always realistic (e.g. when one individual is misidentified for another, or when natural marks or tags are used to identify individuals). Moreover, fitting these models is often computationally inefficient.
This project aims to develop a general, computationally efficient modelling framework to address these issues without relying on strict or unrealistic assumptions. This would not only make CR models more reliable and robust for estimation of key animal population characteristics, but also more accessible to wider scientific community.
Awarded: Carnegie PhD Scholarship
Field: Mathematics & Statistics
University: University of Glasgow